Business analytics (BA) is the practice of iterative, methodical exploration of an organization’s data with emphasis on statistical analysis. Business analytics is used by companies committed to data-driven decision making.
Data analysis is an integral part of research for any organization. Every organization, whether large, medium or small, belonging to any industry in any part of the world, has relevant data that it uses for analytics. How many organizations effectively use that data to drive their business forward is a question worth asking. And whether the data is being correctly utilized or not?
The applications of Business Analytics are numerous. For example, an ecommerce company having a foothold of thousands per day, sells hundreds of products in a day, would have tons of data in record. They can easily analyze the bestselling product, the most frequent buyers, the most successful sale campaigns, and the region from where most customers are from, etc. through the help of this data. It would undoubtedly help them in serving their customers better and making the best and most wanted products available regularly.
But we again come back to effectiveness. Is the analytics giving the organization a desired competitive edge? Are they able to gain timely, market insights?
MIT Sloan Management Review, in collaboration with IBM Institute for Business Value, conducted a survey of more than 3000 business executives from around the world to understand the challenges and opportunities associated with the use of business analytics. One of their key findings was that the top performing organizations use analytics five times more than lower performers.
The study also revealed that the biggest obstacles to analytics adoption are the lack of understanding how to use analytics for the improvement and advancement of the business.
Many businesses use tools like PESTLE and SWOT analysis for better decision making. But aren’t these tools, too, a part of data analysis?
PESTLE is used to identify the political, economic, social, technological, legal and environmental factors that may have an effect on a project and its planning process. This analysis helps in evaluating the risks, threats and opportunities to avoid / exploit in the strategy.
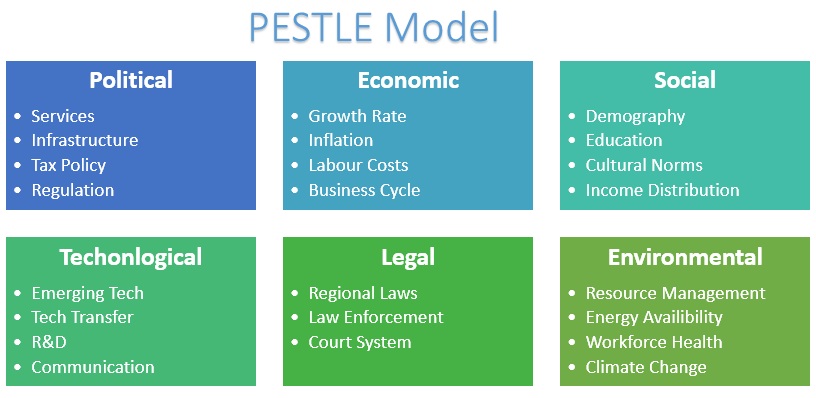
Both of these tools make use of data analytics to help businesses in strategic planning and decision making for the growth of the firm.
When you are a founder or an entrepreneur, you need all the relevant data to back up your goal or vision for your company. You need to know what are getting into.
Tools like PESTLE and SWOT analysis helps the organization in value addition for the betterment. You may ask we did a PESTLE / SWOT analysis when we were growing. I counter. What about now? Is once enough? Or have you stopped growing now?
What do you do when thinking of expansion?
What do you do when considering Return on Investments?
What do you do when strategizing about how to acquire new clients?
What do you do when contemplating where to invest and which areas to drop?
One simple answer – Data Analysis, though it is not as simple to implement.
Data validation is the next step to data analysis. Once you have gathered the relevant data, you need to be sure that it is, in fact, correct. One small mistake can cost you your business.
Since information is constantly being updated, deleted, queried, or moved around, having valid data is a must. According to MarketingSherpa, an average of 2.1% of contact details change every month!
In 2009, Biznology updated the original 2002 study on B2B Data Decay that has been cited by Dun & Bradstreet, Zoom, Jigsaw (now part of Salesforce.com) and others. Here are the results:
- 8% name change (women still do change their name upon marriage/divorce)
- 8% title and/or job function change (changed firm or new job in the same firm)
- 9% address change (changing companies or company/individual’s relocation)
- 9% phone number change (changing companies and company move as above)
- 3% email address change (slightly less than above as some use personal email address)
Upon further analysis they were able to gather a few more data decay statistics. They are:
- 6% of individuals changed companies (new job)
- 2% of individuals changed jobs in their company (promotion or reorganization)
- 6% of the companies changed their name (merger or rebranding)
- 3% of companies moved office locations
Since we all target and sell to individuals and not companies, it is critical to have the individuals’ details and contact information correct.
Many a times, correct data is as much dependent on people as on facts. But people view things differently and thus data validation requires working to different perspectives.
Four of the most common perspectives are:
- The Analytical Perspective – Describing the quantitative value that will be created.
- The Process Perspective – Emphasizing on the execution and implementation.
- The Personal Perspective – How the individual professional is impacted.
- The Strategic Perspective – Focus on the competencies and competitive advantage.
Being able to translate massive amounts of data into real insight is gaining a huge competitive advantage.
Data Analysis without Data Validation is of no use at all. Be it people data, market data, industry data, etc., it is important to have the perfect combination of Data Collection, Validation and Analysis to benefit. All you need is the accurate and relevant data and utilize it effectively; it would automatically add value to your business!
One response
Data validation is the next step to data analysis. Once you have gathered the relevant data, you need to be sure that it is, in fact, correct. One small mistake can cost you your business.